The CRV program includes a number of invited speakers from all over to talk about their research programs targeting computer vision and robotics. Keynote speakers will give long talksto kick off each day. Symposium speakers will give short talks and chair each session. The confirmed speakers are listed below. Details will be udpated later.
CRV 2023 speakers (in alphabetical order) are:
Keynote Speakers
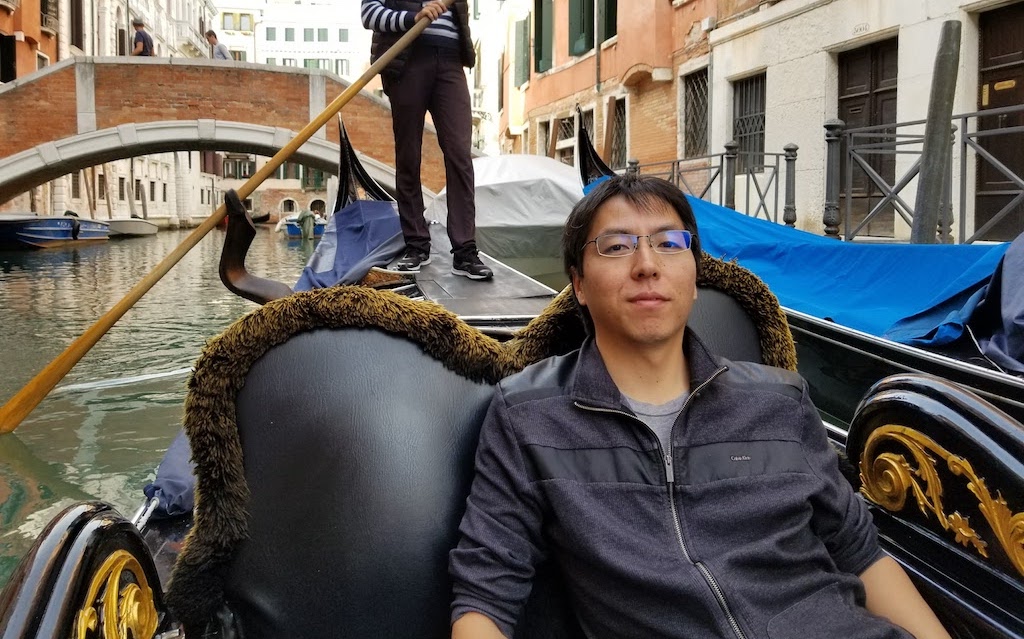
Yasutaka Furukawa
Simon Fraser University
Talk Title: Diffusion Models for Reconstruction, Generation, and Pose Estimation
Abstract
Generative AI has seen a surge in popularity, with Diffusion Models (DMs) being a crucial component of many successful visual content generation techniques. Examples include DALL-E by OpenAI, Imagen by Google, and Stable Diffusion by Stability AI. While DMs are commonly known for their ability to generate content, our research group has discovered that DMs are also highly effective general problem solvers. Specifically, we focus on structured geometry modeling (e.g., CAD models), and have recently made significant strides in vector-graphics floorplan generation, vector-graphics floorplan and HD map reconstruction, and spatial arrangement estimation. With the use of DMs, our system consistently achieves the best performance across all tasks, surpassing existing state-of-the-art methods tailored to specific tasks.Bio
Dr. Yasutaka Furukawa is an associate professor in the School of Computing Science at Simon Fraser University (SFU). Dr. Furukawa's group has made fundamental and practical contributions to 3D reconstruction algorithms, improved localization techniques, and computational architectural modeling. Their open-source software has been widely adopted by tech companies and used in surprising applications such as 3D printing of turtle shells and archaeological reconstruction. Dr. Furukawa received the best student paper award at ECCV 2012, the NSF CAREER Award in 2015, CS-CAN Outstanding Young CS Researcher Award 2018, Google Faculty Research Awards in 2016, 2017, and 2018, and PAMI Longuet-Higgins prize in 2020.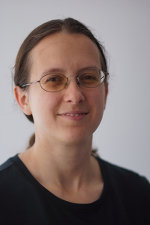
Stefanie Tellex
Brown University
Talk Title: Towards Complex Language in Partially Observed Environments
Abstract
Robots can act as a force multiplier for people, whether a robot assisting an astronaut with a repair on the International Space station, a UAV taking flight over our cities, or an autonomous vehicle driving through our streets. Existing approaches use action-based representations that do not capture the goal-based meaning of a language expression and do not generalize to partially observed environments. The aim of my research program is to create autonomous robots that can understand complex goal-based commands and execute those commands in partially observed, dynamic environments. I will describe demonstrations of object-search in a POMDP setting with information about object locations provided by language, and mapping between English and Linear Temporal Logic, enabling a robot to understand complex natural language commands in city-scale environments. These advances represent steps towards robots that interpret complex natural language commands in partially observed environments using a decision theoretic framework.Bio
Stefanie Tellex is an Associate Professor of Computer Science at Brown University. Her group, the Humans To Robots Lab, creates robots that seamlessly collaborate with people to meet their needs using language, gesture, and probabilistic inference, aiming to empower every person with a collaborative robot. She completed her Ph.D. at the MIT Media Lab in 2010, where she developed models for the meanings of spatial prepositions and motion verbs. Her postdoctoral work at MIT CSAIL focused on creating robots that understand natural language. She has published at SIGIR, HRI, RSS, AAAI, IROS, ICAPs and ICMI, winning Best Student Paper at SIGIR and ICMI, Best Paper at RSS, and an award from the CCC Blue Sky Ideas Initiative. Her awards include being named one of IEEE Spectrum's AI's 10 to Watch in 2013, the Richard B. Salomon Faculty Research Award at Brown University, a DARPA Young Faculty Award in 2015, a NASA Early Career Award in 2016, a 2016 Sloan Research Fellowship, and an NSF Career Award in 2017. Her work has been featured in the press on National Public Radio, BBC, MIT Technology Review, Wired and Wired UK, as well as the New Yorker. She was named one of Wired UK's Women Who Changed Science In 2015 and listed as one of MIT Technology Review's Ten Breakthrough Technologies in 2016.Symposium Speakers
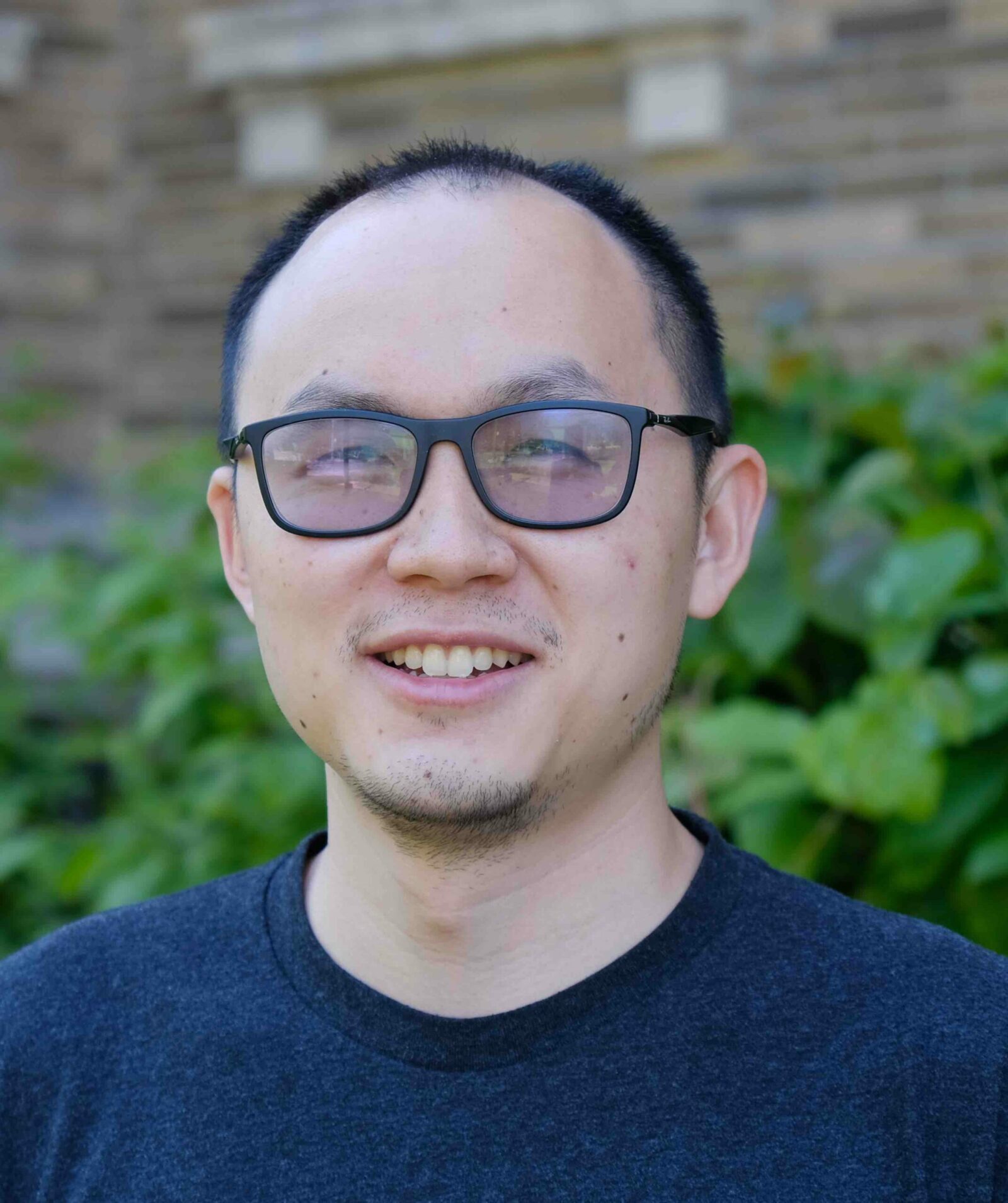
Renjie Liao
University of British Columbia
Talk Title: Specformer: Spectral Graph Neural Networks Meet Transformers
Abstract
Spectral graph neural networks (GNNs) learn graph representations via spectral-domain graph convolutions. However, most existing spectral graph filters are scalar-to-scalar functions, i.e., mapping a single eigenvalue to a single filtered value, thus ignoring the global pattern of the spectrum. Furthermore, these filters are often constructed based on some fixed-order polynomials, which have limited expressiveness and flexibility. To tackle these issues, we introduce Specformer, which effectively encodes the set of all eigenvalues and performs self-attention in the spectral domain, leading to a learnable set-to-set spectral filter. We also design a decoder with learnable bases to enable non-local graph convolution. Importantly, Specformer is equivariant to permutation. By stacking multiple Specformer layers, one can build a powerful spectral GNN. On synthetic datasets, we show that our Specformer can better recover ground-truth spectral filters than other spectral GNNs. Extensive experiments of both node-level and graph-level tasks on real-world graph datasets show that our Specformer outperforms state-of-the-art GNNs and learns meaningful spectrum patterns. Code and data are available at https://github.com/bdy9527/Specformer.Bio
Renjie Liao is an assistant professor at the Department of Electrical and Computer Engineering (ECE) and an associated member of the Department of Computer Science at the University of British Columbia (UBC). He is a faculty member at the Vector Institute and a Canada CIFAR AI Chair. Before joining UBC, he was a Visiting Faculty Researcher at Google Brain, working with Geoffrey Hinton and David Fleet. He received his Ph.D. in CS in 2021 from the University of Toronto under the supervision of Richard Zemel and Raquel Urtasun. During his Ph.D., he worked as a Senior Research Scientist at Uber Advanced Technologies Group. He received an M.Phil. degree in CS in 2015 from the Chinese University of Hong Kong and a B.Eng. degree in Automation in 2011 from Beihang University. He is broadly interested in machine learning and its intersection with computer vision, self-driving, healthcare, and other areas, with a focus on probabilistic and geometric deep learning.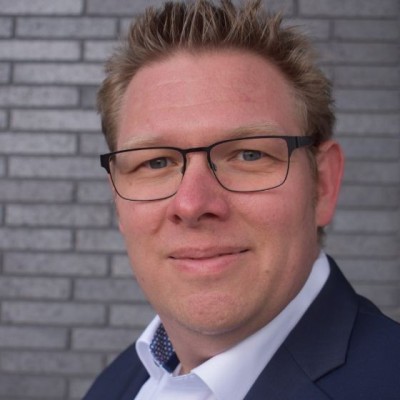
Lueder Kahrs
University of Toronto
Talk Title: Making the Cut Autonomously with Surgical Robot Instruments
Abstract
This talk explores the use of robot cutting techniques with full autonomy and shared control for a future use in surgery. A larger focus is on how computer vision and physics-based simulations can be used to develop suitable assistance methods for physicians. Methods need to be designed to compensate for material deformations or topological changes. Vision-based reinforcement learning is applied to perform cuts autonomously. A shared control framework can give the responsibility partially back to the physicians. The talk will give an literature overview in this domain and show results from the Medical Computer Vision and Robotics (MEDCVR) lab at the University of Toronto.Bio
Lueder A. Kahrs is tenure-stream Assistant Professor in the Department for Mathematical and Computational Sciences at the University of Toronto Mississauga with cross-appointments to the Department of Computer Science and Institute of Biomedical Engineering at the University of Toronto. He founded the Medical Computer Vision and Robotics (MEDCVR) lab that solves challenges of optical, spatial, cognitive or manipulative limits in diagnosis and therapy. Lueder got his PhD (Dr.-Ing.) from Universität Karlsruhe / KIT, Germany in 2009 and his Diplom (M.Sc./B.Sc.) in Physics in 2002 from the University of Bremen, Germany. Before joining UofT, he was senior scientist at the University of Hannover, Germany as well as postdoctoral researcher in Düsseldorf, Germany and Nashville, TN, USA.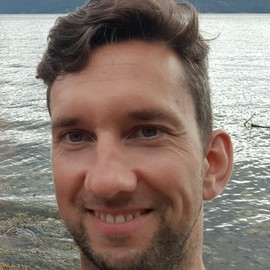
Karsten Kreis
NVIDIA
Talk Title: From Images and Video to 3D Shapes: Content Creation with Diffusion Models
Abstract
Denoising diffusion-based generative models have led to multiple breakthroughs in deep generative learning. In this talk, I will provide an overview over recent works by the NVIDIA Toronto AI Lab on diffusion models and their applications for digital content creation. I will start with a short introduction of diffusion models, recapitulate their mathematical formulation, and briefly discuss how diffusion models have been used for large-scale image and video generation. Then, I will highlight efforts on 3D generative modeling. This includes object-centric 3D synthesis by training diffusion models on geometric shape datasets or leveraging large-scale text-to-image diffusion models as priors for shape distillation.Bio
Karsten Kreis is a senior research scientist at NVIDIA’s Toronto AI Lab. Prior to joining NVIDIA, he worked on deep generative modeling at D-Wave Systems and co-founded Variational AI, a startup utilizing generative models for drug discovery. Before switching to deep learning, Karsten did his M.Sc. in quantum information theory at the Max Planck Institute for the Science of Light and his Ph.D. in computational and statistical physics at the Max Planck Institute for Polymer Research. Currently, Karsten's research focuses on developing novel generative learning methods and on applying deep generative models on problems in areas such as computer vision, graphics and digital artistry, as well as in the natural sciences.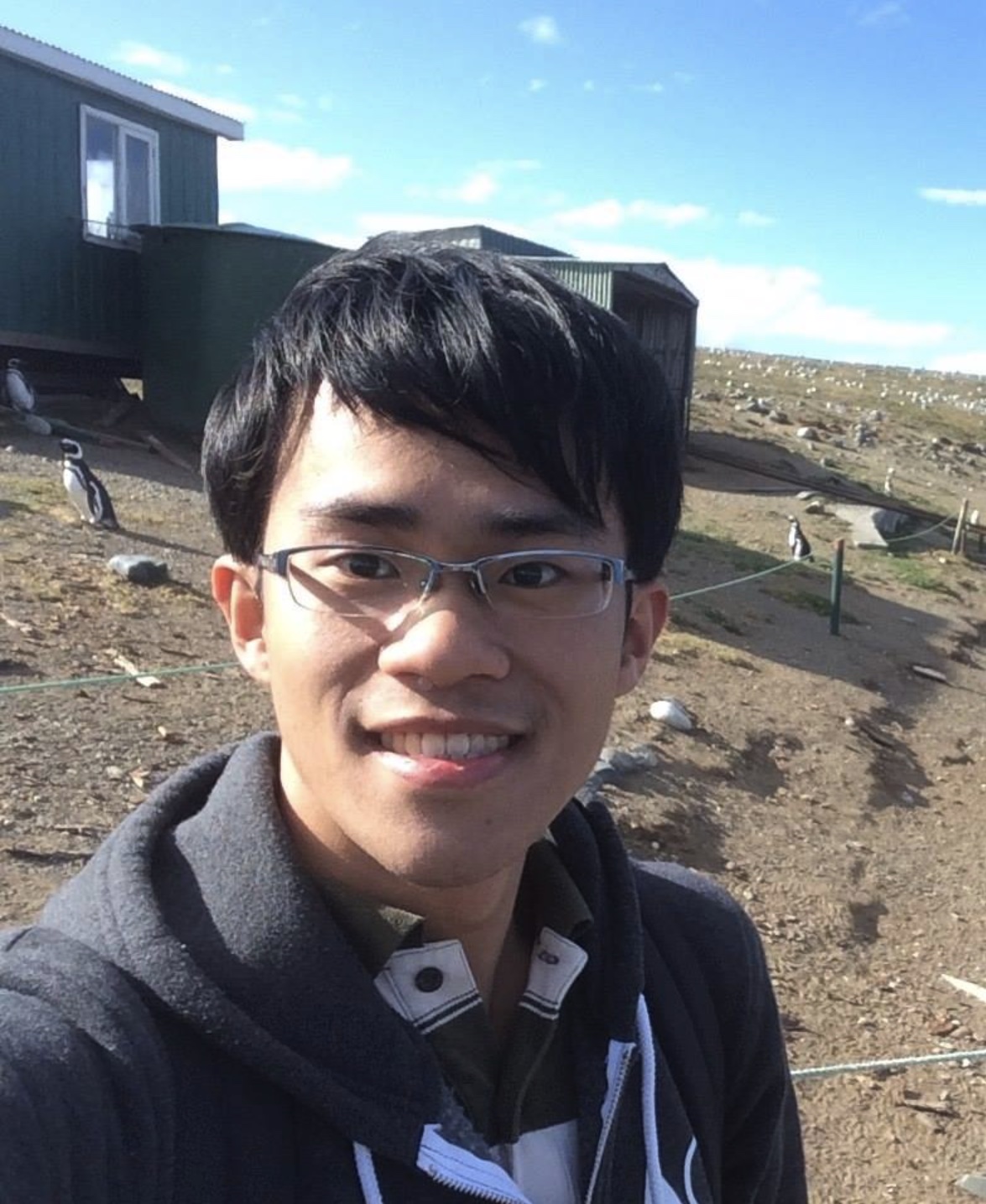
Weicheng Kuo
Google
Talk Title: Exploring image-language pre-training for open-vocabulary object detection
Abstract
Image-language pre-training has become a popular method to learn strong visual representation. However, these models are mostly trained on image-level objectives, and it is non-trivial to adapt them for region-level tasks e.g. detection. In this talk, I'll represent a few of our works on how to use image-language pre-training for open-vocabulary object detection.Bio
Weicheng Kuo is a senior research scientist in Google Brain/Deepmind working on the intersection of computer vision and natural language. His work involves image-language pretraining and object recognition. Before that, he was a research scientist at Google Brain Robotics, where I work on 2D/3D perception for autonomous agents. He received his PhD in Computer Science from UC Berkeley, where he was advised by Prof. Jitendra Malik.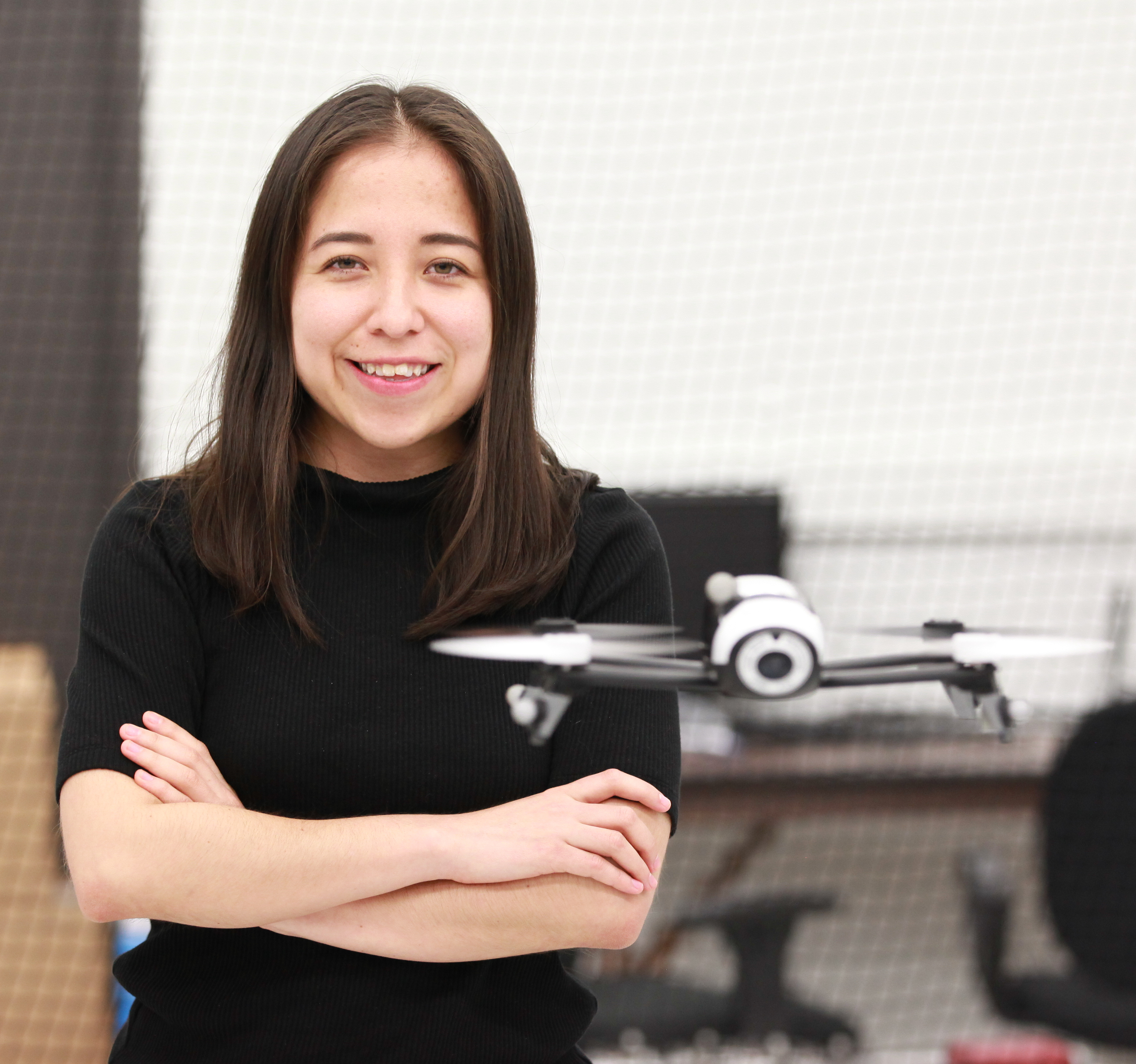
Karime Pereida
Kindred AI
Talk Title: Enabling robots to handle groceries, parcels and apparel
Abstract
Automating fulfillment places robots at the heart of the operation. Robots must reliably and quickly complete tasks in order to meet their expected performance. Some of the main challenges arise from the wide range of objects robots are expected to manipulate. In this talk, I will present different learning-based approaches that Ocado Technology is taking to enable robots to accurately identify and quickly manipulate objects.Bio
Karime Pereida is a Machine Learning and Controls Researcher at Ocado Technology. Her current research focuses on modeling and control of robotic arms to achieve dexterous manipulation of a wide range of objects. Previously she was a Postdoctoral Fellow at the University of Toronto Institute for Aerospace Studies, where she also received her PhD under the supervision of Angela Schoellig. Before that she received her Bachelor’s degree in Mechatronics engineering from Tecnológico de Monterrey, México and her Master’s from the University of New South Wales, Australia.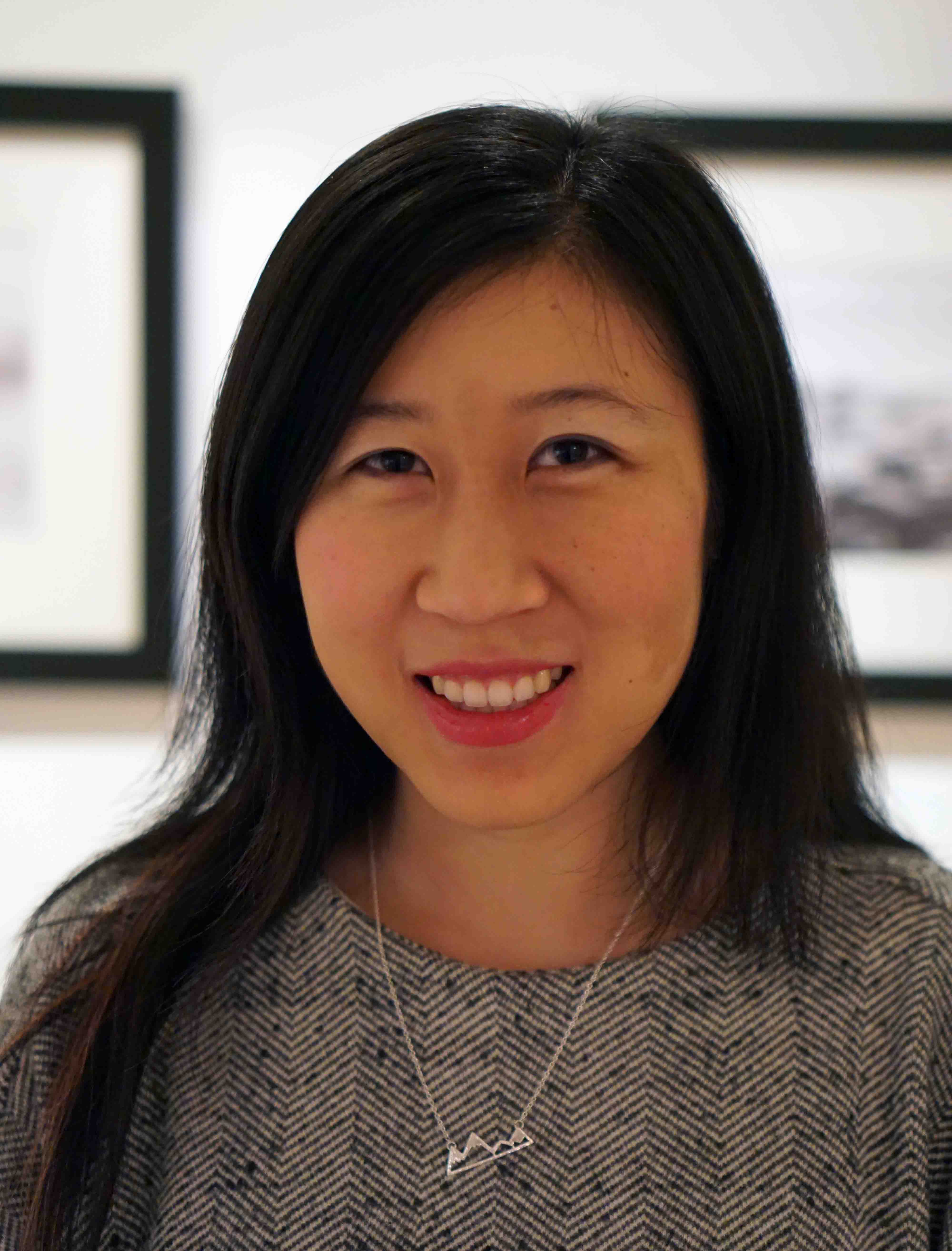