The CRV program includes a number of invited speakers from all over to talk about their research in computer vision and robotics. The confirmed speakers for CRV 2025 are listed below. Details forthcoming.
Keynote Speakers
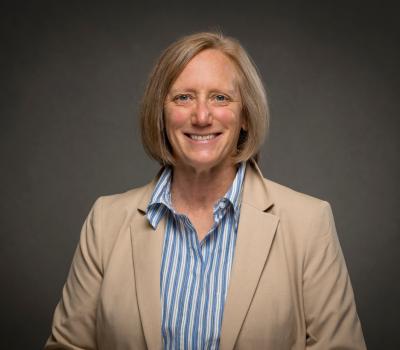
Julie A. Adams
Oregon State University
Talk Title: The Human-Robot Ratio (m:N) Theory: Limitations and Considerations
Abstract
The traditional human-to-robot ratio, or m:N theory states that the number of robots limits humans ability to manage and maintain overall team performance. This theory was developed primarily based on ground robot capabilities 10-15 years ago. While some traditional m:N limitations persist, both applied research and commercial systems debunk this traditional theory, particularly for very large numbers of robots (m<<N). This keynote will discuss the limitations of the theory, provide evidence that contradicts the theory, and discuss human factors aspects that will have an impact on the number of robots a single human can safely deploy. Results and examples will include simulated large autonomous uncrewed aircraft with associated necessary interactions with air traffic control, heterogeneous swarms deployed in urban environments, and commercial delivery uncrewed aircraft.Bio
Dr. Adams is the founder of the Human-Machine Teaming Laboratory and the Associate Director of Research of the Collaborative Robotics and Intelligent Systems (CoRIS) Institute. Adams has focused on human-machine teaming and distributed artificial intelligence for thirty-five years. Throughout her career she has focused on unmanned systems, but also focused on crewed civilian and military aircraft at Honeywell, Inc. and commercial, consumer and industrial systems at the Eastman Kodak Company. Her research, which is grounded in robotics applications for domains such as first response, archaeology, oceanography, and the U.S. military, focuses on distributed artificial intelligence, swarms, robotics and human-machine teaming. Dr. Adams is an NSF CAREER award recipient, a Human Factors and Ergonomics Society Fellow as well as a member of the National Academies Board on Army Research and Development and the DARPA Information Science and Technology Study Group.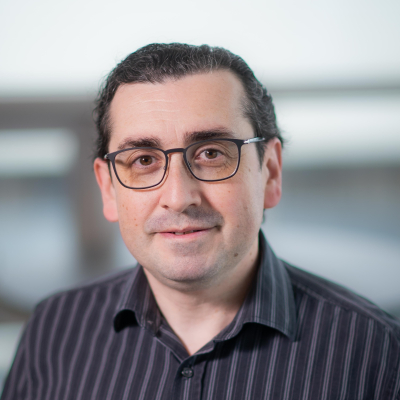
Leonid Sigal
University of British Columbia
Talk Title: The Curious Case of Foundational and VLM Models
Abstract
The capabilities and the use of foundational (FM) and vision-language (VLM) models in computer vision have exploded over the past few years. This has led to a broad paradigm shift in the field. In this talk I will focus on the recent work from my group that navigates this quickly evolving research landscape. Addressing challenges such as building foundational models with better generalization, increasing their context length, adopting them to ever evolving task landscape and routing information among them for more complex reasoning visual problems. I will also discuss some curious benefits and challenges of working with such models, including emergent (localization) capabilities and in-consistency in their responses.Bio
Prof. Leonid Sigal is a Professor at the University of British Columbia (UBC). He was appointed CIFAR AI Chair at the Vector Institute in 2019 and an NSERC Tier 2 Canada Research Chair in Computer Vision and Machine Learning in 2018. Prior to this, he was a Senior Research Scientist, and a group lead, at Disney Research. He completed his Ph.D at Brown University in 2008; received his B.Sc. degrees in Computer Science and Mathematics from Boston University in 1999, his M.A. from Boston University in 1999, and his M.S. from Brown University in 2003. Leonid's research interests lie in the areas of computer vision, machine learning, and computer graphics; with the emphasis on approaches for visual and multi-modal representation learning, recognition, understanding and generative modeling. He has won a number of research awards, including Killam Accelerator Fellowship in 2021 and has published over 100 papers in venues such as CVPR, ICCV, ECCV, NeurIPS, ICLR, and Siggraph.Symposium Speakers
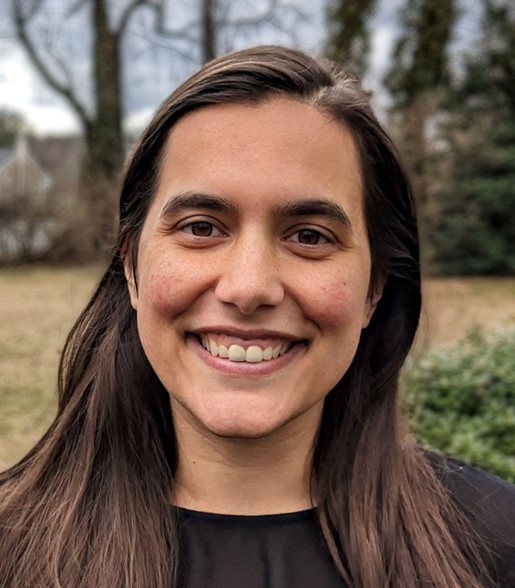
Bernadette Bucher
University of Michigan
Talk Title: Building Visual Representations with Foundation Models for Mobile Manipulation
Abstract
Rapid improvements over the past few years in computer vision have enabled high performing geometric state estimation on moving camera systems in day-to-day environments. Furthermore, recent substantial improvements in language understanding and vision-language grounding have enabled rapid advancements in semantic scene understanding. In this presentation, I will demonstrate how we can build visual representations from these foundational vision-language models to enable new robotic capabilities in navigation, manipulation, and mobile manipulation. I will also discuss new robotics research directions opened up by these advancements in vision-language understanding.Bio
Bernadette Bucher is an Assistant Professor in the Robotics Department at University of Michigan. She leads the Mapping and Motion Lab which focuses on learning interpretable visual representations and estimating their uncertainty for use in robotics, particularly mobile manipulation. Her work has been recognized by a Best Paper Award in Cognitive Robotics at ICRA 2024 and is funded by NASA and General Motors. Before joining University of Michigan this fall, she was a research scientist at the Boston Dynamics AI Institute, a senior software engineer at Lockheed Martin Corporation, and an intern at NVIDIA Research. She earned her PhD from University of Pennsylvania and bachelor’s and Masters degrees from University of Alabama.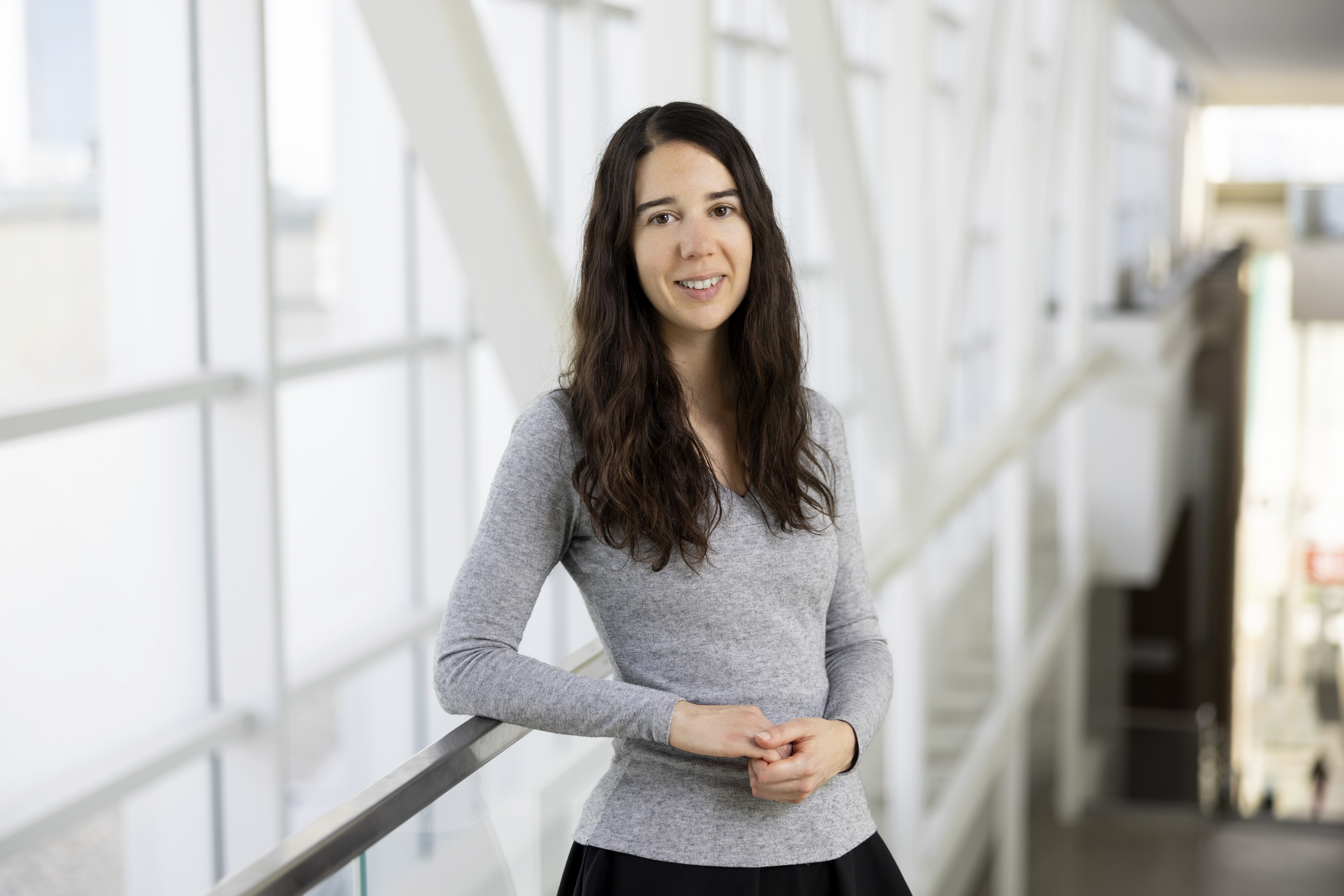
Marie Charbonneau
University of Calgary
Talk Title: Contact-based interaction for better human-robot collaborations
Abstract
Touch is a central component of humans' interactions with others and with the world. While robots are increasingly being developed to work alongside people, their capacity to interact with humans through touch is yet underdeveloped. This talk will explore why this may be the case, why it matters, and recent research at the Waterloo RoboHub and Calgary Human-Robot Collaboration lab towards making robots more physically interactive.Bio
Dr. Marie Charbonneau works to make human-robot interactions safe, comfortable, and intuitive. Dr. Charbonneau joined the University of Calgary as Assistant Professor in September 2021, following post-doctoral work in humanoid robotics at the University of Waterloo and a PhD in Advanced and Humanoid Robotics from the Istituto Italiano di Tecnologia and the Università Degli Studi di Genova. Dr. Charbonneau’s work in whole-body control regulates the forces between robots and their environment, towards ensuring respectful and reliable interactions with people. For instance, Dr. Charbonneau has programmed a humanoid robot to waltz with human partners, and currently works on improving a robot's awareness of and response to physical contacts.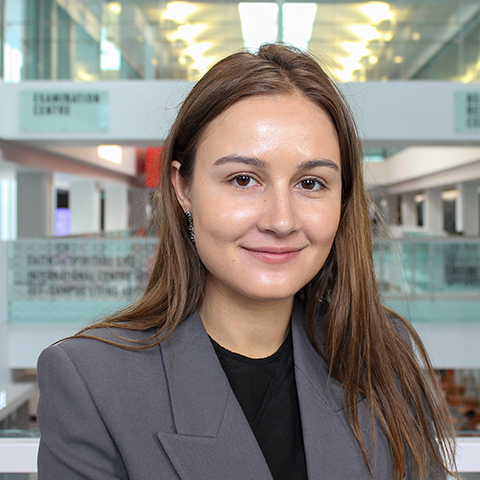
Melissa Greeff
Queen's University
Talk Title: Robots Helping Robots: Enhancing Cross-Modal Interactions Between Aerial, Ground, and Surface Vessel Robots
Abstract
Single aerial robot systems can achieve high speed flight in challenging GPS-denied conditions enabling remote surveillance, package delivery and infrastructure inspection. However, we can further enhance robot operability in diverse environments (from air to land to marine) by augmenting the autonomous capabilities of single aerial, ground or surface vessels through cross-modal interactions. In this talk, we will discuss two different applications that benefit from cross-modality. Firstly, we explore how to leverage aerial robot imagery to enable GPS-denied, zero-shot autonomous navigation for ground vehicles in untraversed environments. Secondly, we explore how to co-ordinate autonomous aerial and surface vessels to enable the landing of aerial vehicles on surface vessels to recharge in remote marine or limnology applications. This is done by accommodating spatial and temporal uncertainties in the waves that can make landing challenging. These preliminary technologies have the potential to enable more persistent operation of robots in diverse environments.Bio
Dr Melissa Greeff is an assistant professor in Electrical and Computer Engineering at Queen’s University. She is an Ingenuity Labs Robotics and AI Institute Member and a Faculty Affiliate at the Vector Institute for Artificial Intelligence. She leads Robora Lab. Her research interests include aerial robots, vision-based navigation, and safe learning-based control. She has published in various international robotics and control systems venues including IEEE Robotics and Auto. Letters, Annual Review of Control, Robotics, and Autonomous Systems, ICRA, IROS and CDC. She has helped co-organize various workshops on safe robot learning and benchmarking at various international conferences. Her research is supported by NSERC, CFI, MITACs, the Department of National Defense (DND) and various industry collaborators. Dr. Greeff ‘s expertise is in building autonomous aerial systems including conducting field trials at various locations across Canada. She was listed as one of 50 women in robotics you need to know about in 2023 by the Women in Robotics organization.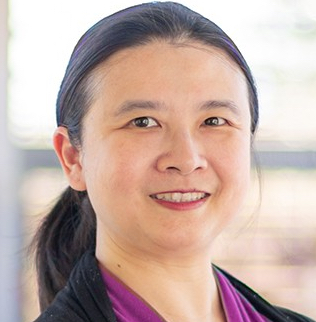
Angel Chang
Simon Fraser University | CIFAR AI Chair at AMII
Talk Title:
Abstract
Bio
Dr. Angel Chang is an Associate Professor at Simon Fraser University. She was previously a visiting research scientist at Facebook AI Research and a research scientist at Eloquent Labs, where she worked on dialogue systems. Dr. Chang earned her Ph.D. in Computer Science from Stanford University, where she was a member of the Natural Language Processing Group under the supervision of Professor Chris Manning. Her research lies at the intersection of language, 3D vision, and embodied AI, with a focus on connecting natural language to 3D representations of shapes and scenes. She is particularly interested in grounding language for embodied agents operating in indoor environments. Dr. Chang has developed methods for synthesizing 3D scenes and shapes from text and contributed to the creation of influential datasets for 3D scene understanding. Her broader interests include the semantics of shapes and scenes, common sense knowledge representation and acquisition, and reasoning using probabilistic models.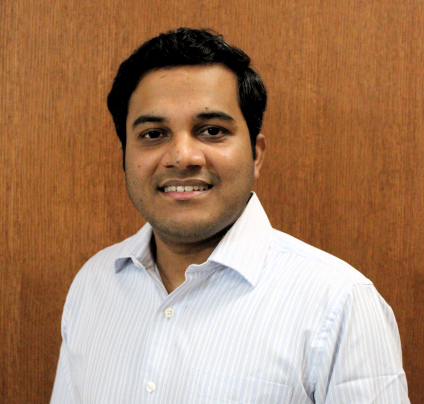
Mrigank Rochan
University of Saskatchewan
Talk Title: Advancing Video Abstraction with Deep Learning
Abstract
As video data continues to grow exponentially in volume and complexity, the development of intelligent systems to manage and summarize videos has become a pressing need. Video abstraction, a key task in computer vision and video understanding, aims to create a short, informative visual summary of a video, enabling users to quickly gain valuable insights about the video without watching it entirely. With applications spanning entertainment, sports, surveillance, healthcare, and video search, this technology has the potential to transform how we interact with video content and unlock the full potential of video data. In this talk, I will discuss our recent research and innovative solutions leveraging deep learning to advance the state of the art in video abstraction.Bio
Dr. Mrigank Rochan is an Assistant Professor in the Department of Computer Science at the University of Saskatchewan, where he leads a research group focusing on computer vision and deep learning. Prior to this, he was a Senior Researcher with the Autonomous Driving Perception team at Huawei Noah's Ark Lab in Toronto. He earned his PhD from the University of Manitoba, and his doctoral thesis was awarded the 2020 Canadian Image Processing and Pattern Recognition Society (CIPPRS) John Barron Doctoral Dissertation Award, a national award presented annually to the top PhD thesis in computer or robot vision in Canada. His research has been published in top-tier computer vision and robotics venues, including CVPR, ICCV, ECCV, ICRA, and TPAMI. Dr. Rochan’s research is currently supported by the University of Saskatchewan, Google, and NSERC.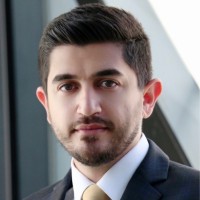