The CRV program includes a number of invited speakers from all over to talk about their research programs targeting computer vision and robotics. Keynote speakers will give long talksto kick off each day. Symposium speakers will give short talks and chair each session. CRV 2021 speakers are:
Keynote Speakers
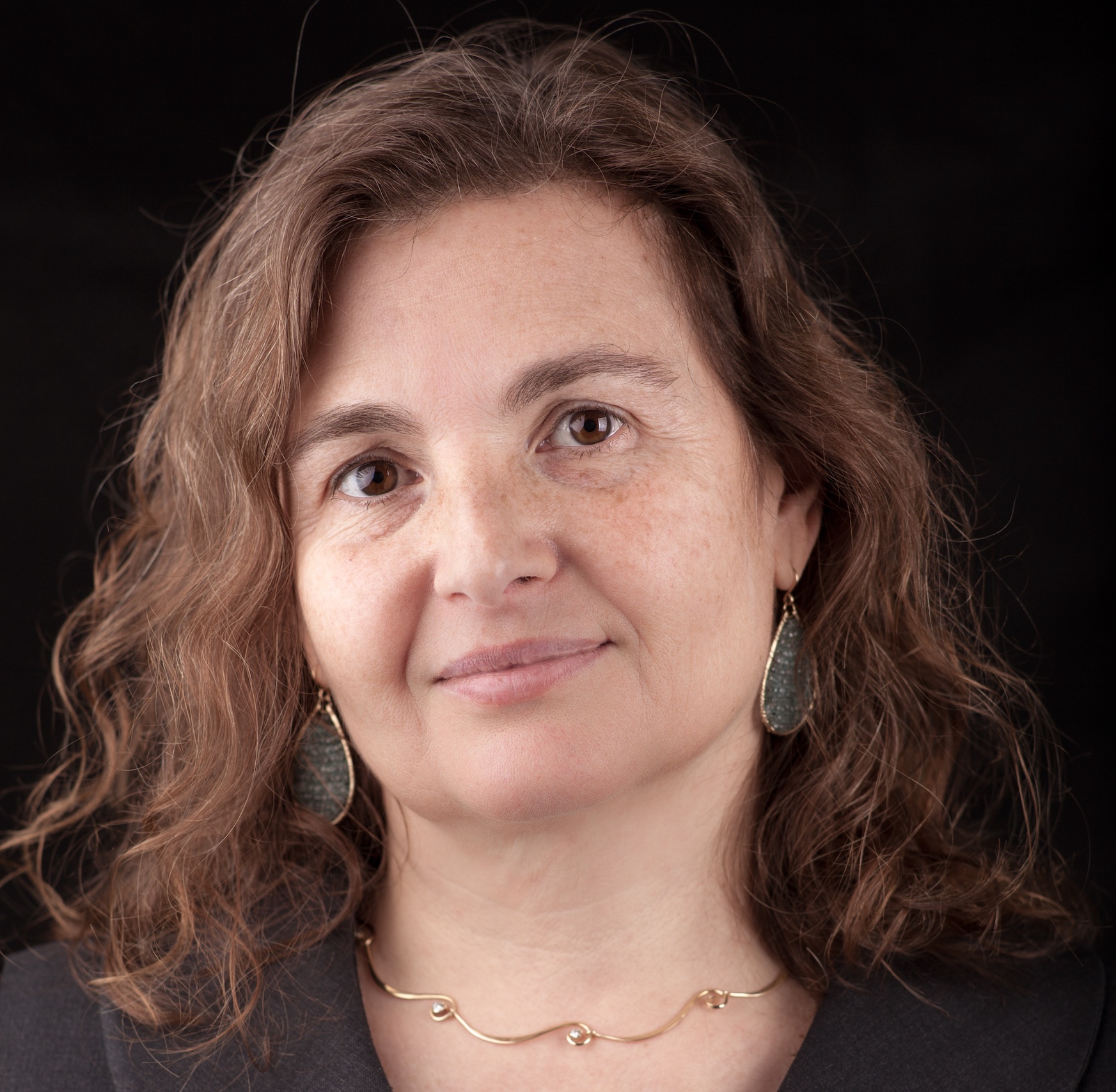
Daniela Rus
MIT CSAIL
Talk Title: Learning Risk and Social Behavior in Mixed Human-Autonomous Vehicles Systems
Abstract
Deployment of autonomous vehicles (AV) on public roads promises increases in efficiency and safety, and requires intelligent situation awareness. We wish to have autonomous vehicles that can learn to behave in safe and predictable ways, and are capable of evaluating risk, understanding the intent of human drivers, and adapting to different road situations. This talk describes an approach to learning and integrating risk and behavior analysis in the control of autonomous vehicles. I will introduce Social Value Orientation (SVO), which captures how an agent’s social preferences and cooperation affect interactions with other agents by quantifying the degree of selfishness or altruism. SVO can be integrated in control and decision making for AVs. I will provide recent examples of self-driving vehicles capable of adaptation.Bio
Daniela Rus is the Andrew (1956) and Erna Viterbi Professor of Electrical Engineering and Computer Science, Director of the Computer Science and Artificial Intelligence Laboratory (CSAIL) at MIT, and Deputy Dean of Research in the Schwarzman College of Computing at MIT. Rus' research interests are in robotics and artificial intelligence. The key focus of her research is to develop the science and engineering of autonomy. Rus is a Class of 2002 MacArthur Fellow, a fellow of ACM, AAAI and IEEE, a member of the National Academy of Engineering, and of the American Academy of Arts and Sciences. She is a senior visiting fellow at MITRE Corporation. She is the recipient of the Engelberger Award for robotics. She earned her PhD in Computer Science from Cornell University.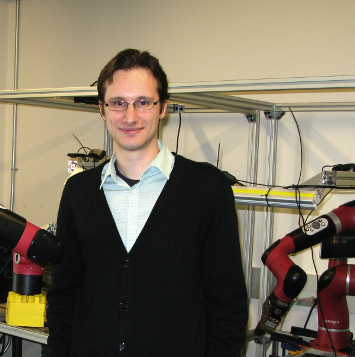
Sergey Levine
UC Berkeley
Talk Title: Generalization in Data-Driven Control
Abstract
Current machine learning methods are primarily deployed for tackling prediction problems, which are almost always cast as supervised learning tasks. Despite decades of advances in reinforcement learning and learning-based control, the applicability of these methods to domains that require open-world generalization -- autonomous driving, robotics, aerospace, and other applications, -- remain challenging. Realistic environments require effective generalization, and effective generalization requires training on large and diverse datasets that are representative of the likely test-time scenarios. I will discuss why this poses a particular challenge for learning-based control, and present some recent research directions that aim to address this challenge. I will discuss how offline reinforcement learning algorithms can make it possible for learning-based control systems to utilize large and diverse real-world datasets, how the use of diverse data can enable robotic systems to navigate real-world environments, and how multi-task and contextual policies can enable broad generalization to a range of user-specified goals.Bio
Sergey Levine received a BS and MS in Computer Science from Stanford University in 2009, and a Ph.D. in Computer Science from Stanford University in 2014. He joined the faculty of the Department of Electrical Engineering and Computer Sciences at UC Berkeley in fall 2016. His work focuses on machine learning for decision making and control, with an emphasis on deep learning and reinforcement learning algorithms. Applications of his work include autonomous robots and vehicles, as well as computer vision and graphics. His research includes developing algorithms for end-to-end training of deep neural network policies that combine perception and control, scalable algorithms for inverse reinforcement learning, deep reinforcement learning algorithms, and more.Symposium Speakers
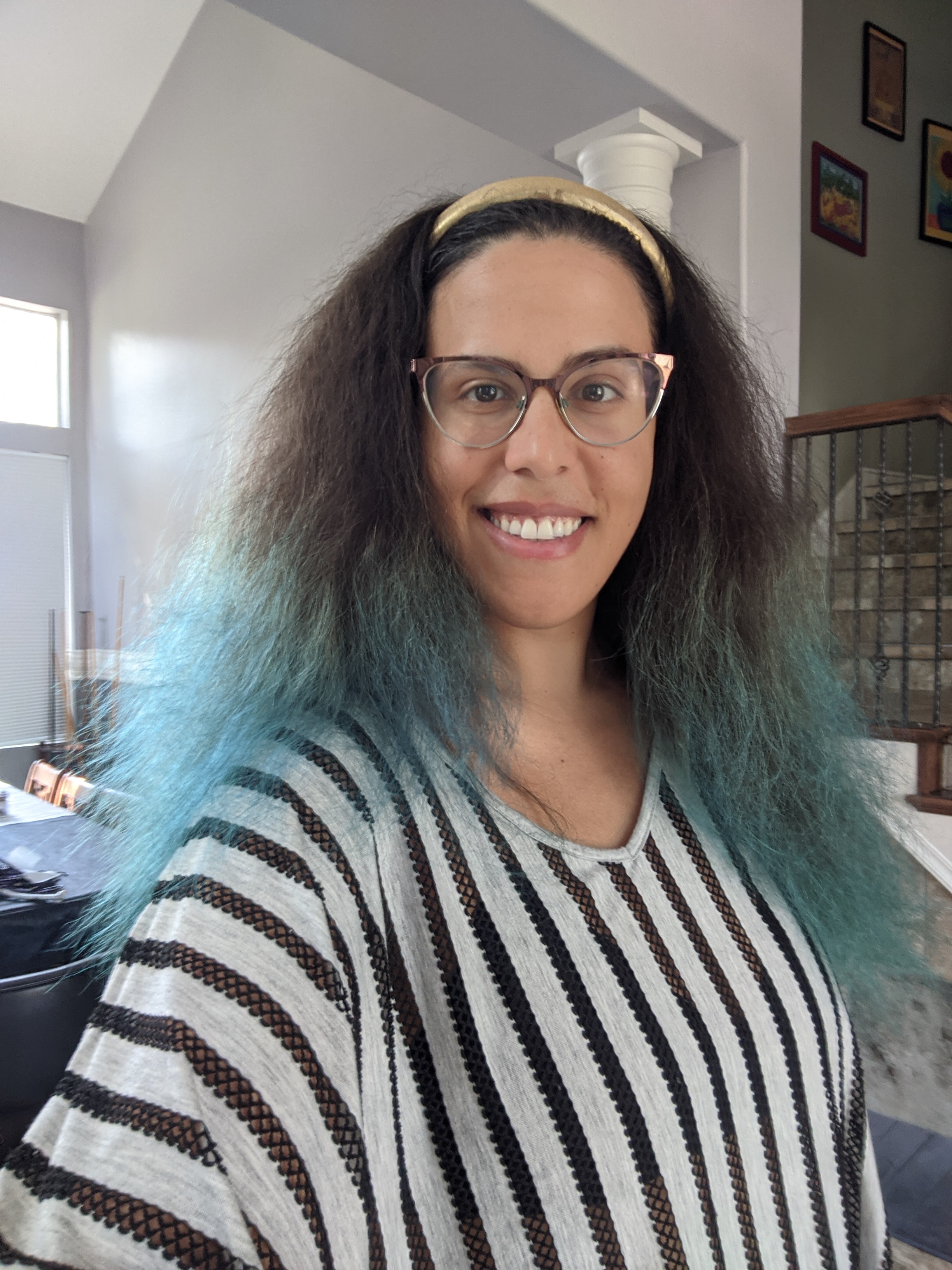
Ariel Anders
robust.ai
Talk Title: Robot manipulation without perception
Abstract
A crucial challenge in robotics is achieving reliable results in spite of sensing and control uncertainty. During my PhD I explored the conformant planning approach to robot manipulation. In particular, pushing multiple planar objects simultaneously to achieve a specified arrangement without external sensing. In this talk, I will share some insights on developing a hybrid model to leverage what we know and learn what we don’t.Bio
Ariel Anders is a black feminist roboticist who enjoys spending time with her family and artistic self-expression. Anders is the first roboticist hired at Robust.AI, an early stage robotics startup building the world’s first industrial grade cognitive engine. Anders received a BS in Computer Engineering from UC Santa Cruz and her Doctorate in Computer Science from MIT, where she taught project-based collaborative robotics courses, developed an iOS app for people with vision impairment, and received a grant to install therapy lamps across campus. Her research focused on reliable robotic manipulation with the vision of enabling household helpers.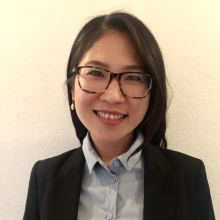
Hsiu-Chin Lin
McGill University
Talk Title: A generic model-based control framework in unstructured environments
Abstract
One key aspect of integrating robots into unstructured environments is that robots need to respond and adapt to unexpected disturbances. In this talk, I will summarize some of my work on optimizing the controller with respect to external forces. I will also discuss my work on the estimation of movement constraints imposed by the tasks or the environment for motion adaptation. The applications were verified on both manipulation and locomotion on real robotic platforms.Bio
Hsiu-Chin Lin is an Assistant Professor in the Computer Science and the Department of Electrical and Computer Engineering at McGill University. Her research spans model-based motion control, optimization, and machine learning for motion planning. She is particularly interested in adapting robot motion in dynamic environments for manipulators and quadruped robots. Prior to McGill, she was a Research Associate at the University of Edinburgh and the University of Birmingham. She received her Ph.D. from the University of Edinburgh for her work on robot learning.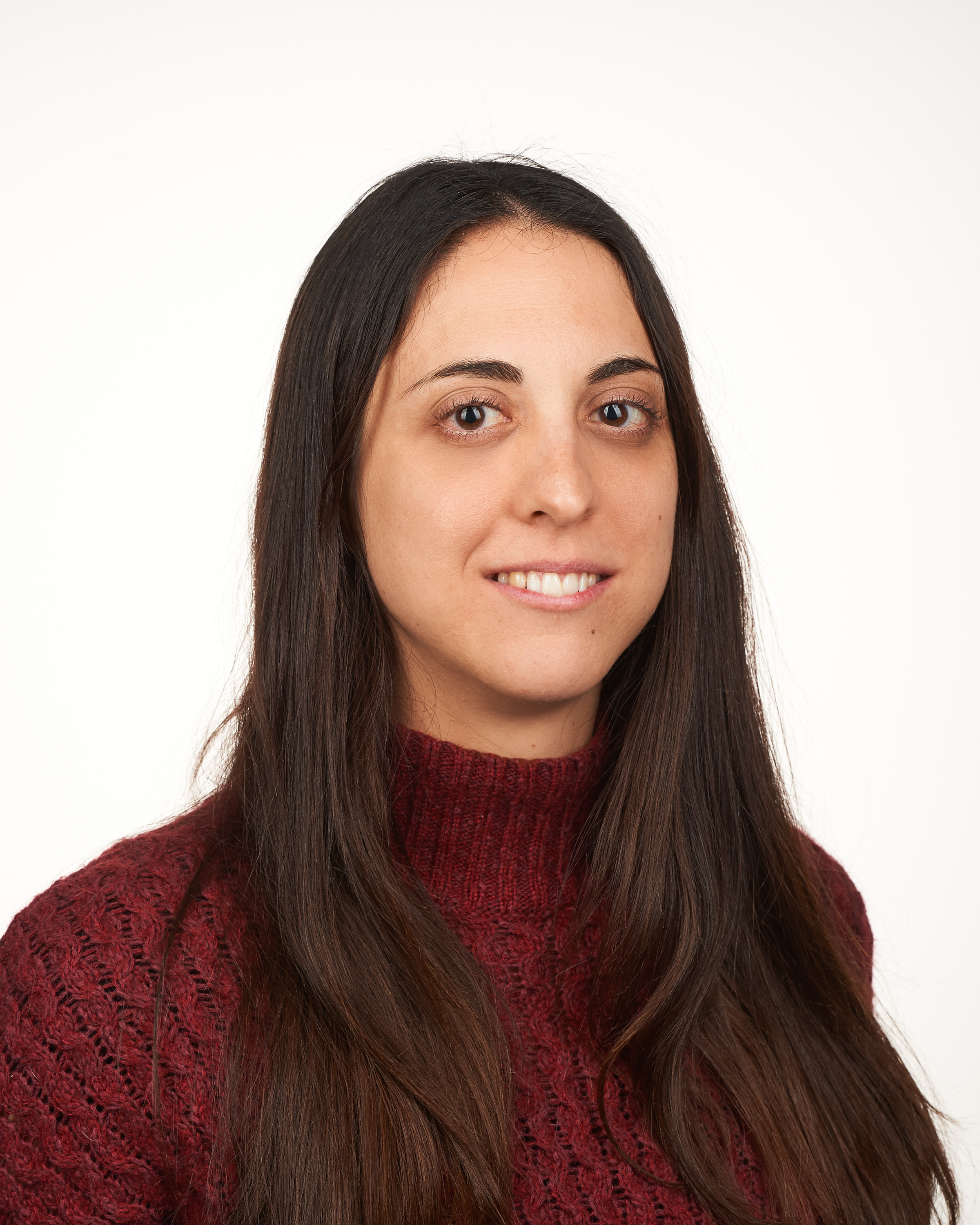
Adriana Romero Soriano
Facebook AI Research
Talk Title: Filling in the blanks with active acquisition
Abstract
As humans we can never fully observe the world around us and yet we are able to build remarkably useful models of it from our limited sensory data. Machine learning systems are often required to operate in a similar setup, that is the one of inferring unobserved information from the observed one. Partial observations entail data uncertainty, which may hinder the quality of the model predictions. In this talk, I will discuss two strategies to mitigate this problem: (1) leveraging the complementarity of different data modalities, and (2) actively acquiring additional information from the same data modality.Bio
Adriana Romero-Soriano is a research scientist at Facebook AI Research and an adjunct professor at McGill University. Her research focuses on developing models and algorithms that are able to learn from multi-modal and real world data, understand and reason about conceptual relations, and recognize their uncertainties, while addressing impactful problems. She completed her postdoctoral studies in 2017 at Mila, where she was advised by Prof. Yoshua Bengio. Her postdoctoral research revolved around deep learning techniques to tackle biomedical challenges, such as the ones posed by multi-modal data, high dimensional data and graph structured data. She received her Ph.D. from University of Barcelona in 2015 with a thesis on assisting the training of deep neural networks with applications to computer vision, advised by Dr. Carlo Gatta.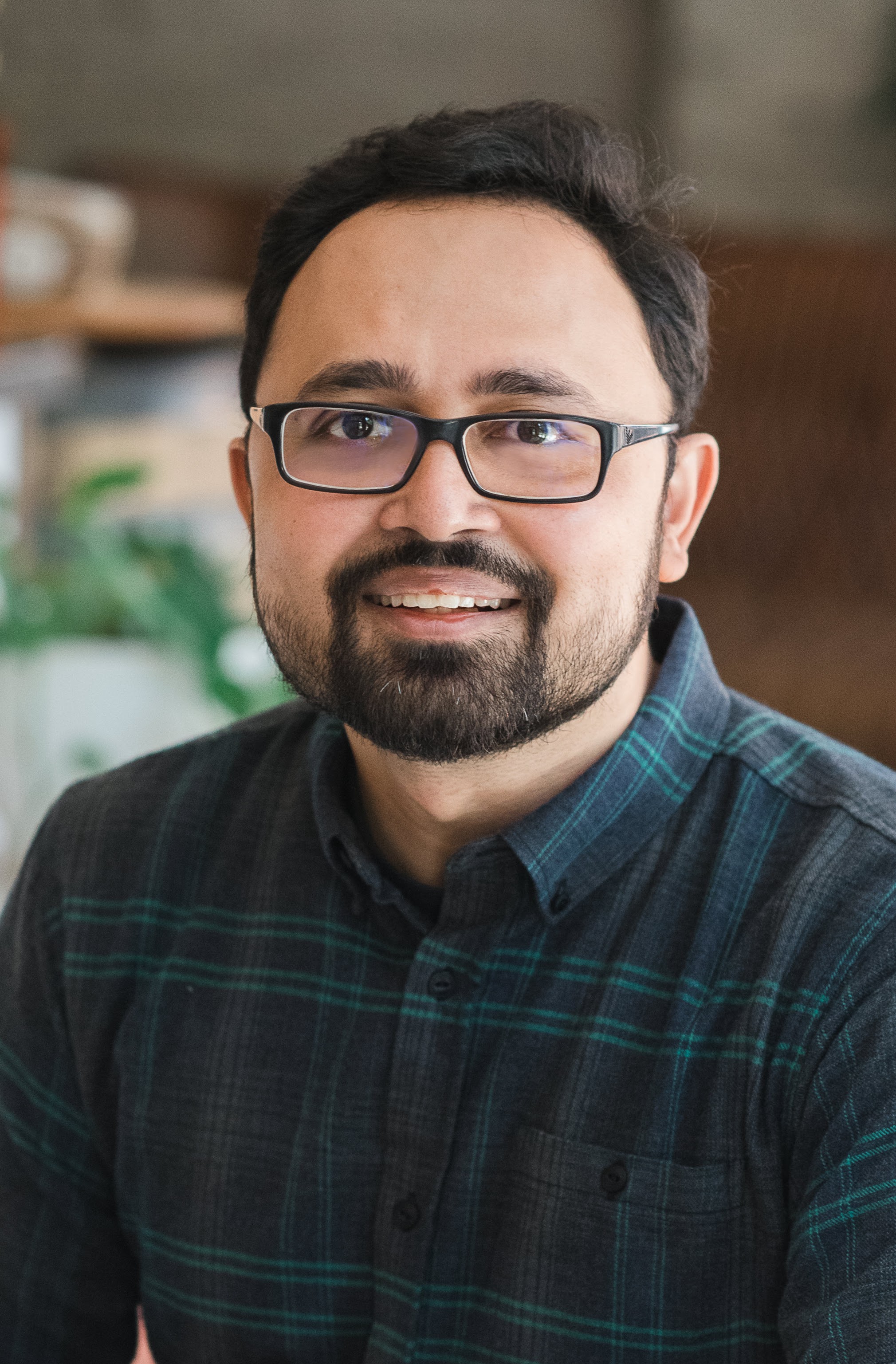
Rupam Mahmood
University of Alberta
Talk Title: End-to-end continual RL with robots
Abstract
A longstanding goal in reinforcement learning (RL) research is to develop an agent that learns continually and end-to-end. Much of this research can be pursued in isolation from the real world using simulated environments. However, the outcomes of this line of research do not appear to be ready for robotics. In this talk, I present the view of adopting physical robotic environments as a way of pursuing and overcoming the challenges of end-to-end continual RL. I argue that many shortcomings of RL become most apparent in physical robotic environments. I present some of these challenges, which would require directed efforts in deep RL research and working on physical environments.Bio
Rupam Mahmood is a Canada CIFAR AI Chair at Alberta Machine Intelligence Institute (Amii) and a faculty member of the Department of Computing Science at the University of Alberta, where he directs the Reinforcement Learning and Artificial Intelligence (RLAI) laboratory. He works in the areas intersecting reinforcement learning and robotics and develops learning systems and constructive learning mechanisms for continually learning robots. Prior to joining UAlberta, Rupam was the Lead of the AI Research team at Kindred AI, which provides robotic solutions for unstructured environments such as the warehouses of Gap Inc. At Kindred AI, Rupam created SenseAct, the first open-source toolkit and benchmark task suite for general-purpose real-time learning with different physical robots. During his graduate studies, he developed solutions to some long-standing problems with step-size adaptation, online representation search, and off-policy learning, a class of techniques for learning knowledge representations in a counter-factual and computationally scalable manner.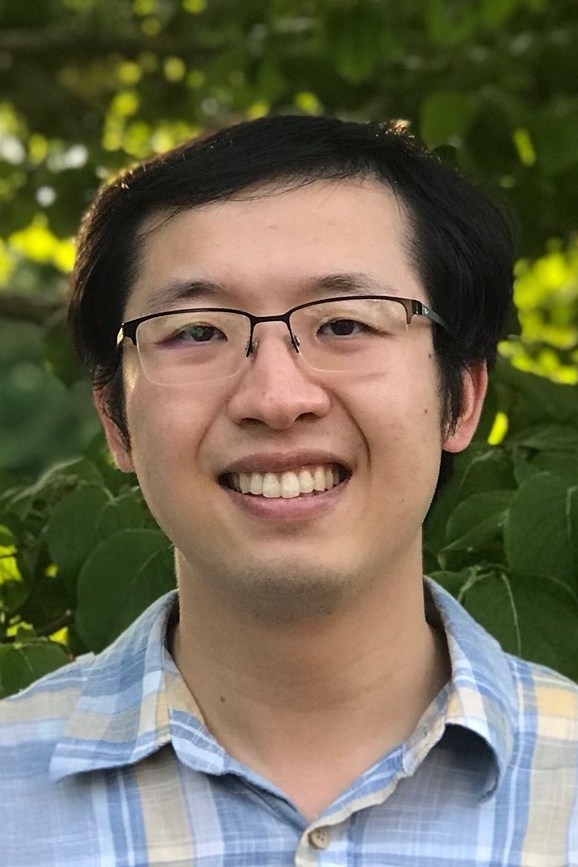
Ke Li
Simon Fraser University
Talk Title: Worry-Free Image Synthesis without Mode Collapse
Abstract
Generative adversarial nets (GANs) are the workhorse of deep learning-based image synthesis methods. In practice, however, they suffer from the well-documented problem of mode collapse and effectively ignore arbitrary subsets of the training data. This problem has proven to be difficult to solve and has persisted through the many successive variants of GANs. I will illustrate why mode collapse happens fundamentally and show how to overcome it, which forms the basis of a new method known as Implicit Maximum Likelihood Estimation (IMLE). In the conditional setting, IMLE overcomes the tendency of GANs to ignore the latent noise and can generate alternative versions of images that are unobserved.Bio
Ke Li is an Assistant Professor in the School of Computing Science at Simon Fraser University. He is interested in a broad range of topics in machine learning, computer vision, NLP and algorithms and has worked on generative modelling, nearest neighbour search and Learning to Optimize. He is particularly passionate about tackling long-standing fundamental problems that cannot be tackled with a straightforward application of conventional techniques. He was previously a Member of the Institute for Advanced Study (IAS), and received his Ph.D. from UC Berkeley and B.Sc. from the University of Toronto.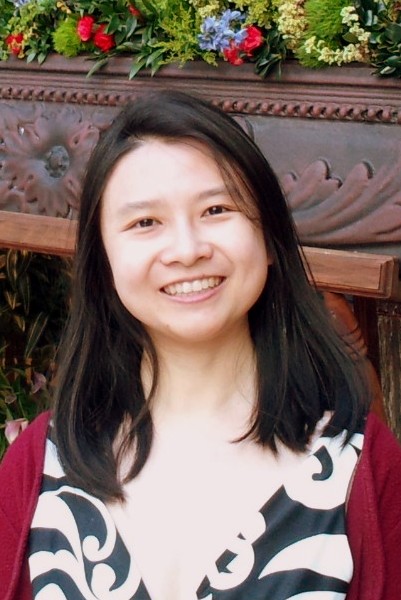